MSU Video Codecs Comparison 2021 Part 2: Subjective
Sixteen Annual Video Codecs Comparison by MSU
|
|||||||||
![]() |
![]() |
![]() |
![]() |
||||||
compression.ru |
Lomonosov Moscow State University (MSU) Graphics and Media Lab |
Dubna International
State University |
Institute for Information Transmission Problems, Russian Academy of Science |
News
- 23.11.2021 Report release
Navigation
Results
- The places below are given only for quality scores, not taking encoding speed into account
Slow (1 fps) | Fast (30 fps) | |||||||||||||
Best quality (YUV-Subjective) |
|
|
For subjective quality measurements we used Subjectify.us crowdsourcing platform. We involved more than 10,800+ participants
Download and buy report
Free | Enterprise | |||
Number of test sequences | 3 of 15 (only Universal use case and only 8-bit content) | All 15 sequences for all use cases | ||
Test video descriptions | ||||
Basic codec info | ||||
Metric: YUV-Subjective, YUV-SSIM, VMAF (overall results) | ||||
Other objective metrics (Y-VMAF(0.6.1 for 4K), Y-SSIM, U-SSIM, V-SSIM, YUV-PSNR, Y-PSNR, U-PSNR, V-PSNR) | ||||
Test videos download | ||||
Encoders presets description | ||||
PDF report | 67 pages | 80 pages | ||
HTML report | 30 interactive charts | 3000+ interacive charts | ||
Price | Free | 950 USD | ||
Download/Buy |
PDF & HTML reports (ZIP)
|
You will receive enterprise versions of all 2021 reports (FullHD, Subjective, 4K)
|
Participated codecs
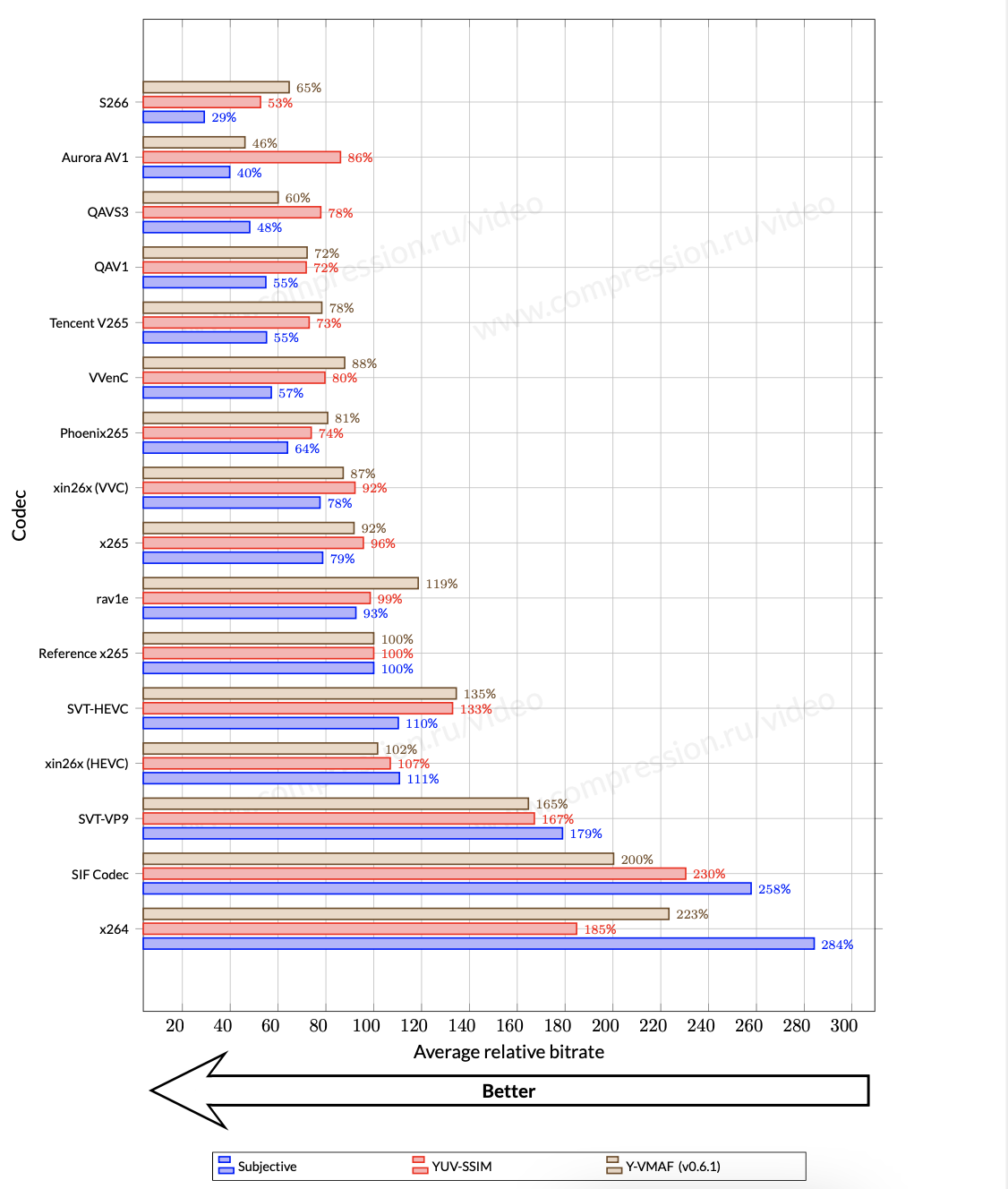
Codec name | Use cases | Standard | Version | |
1 |
Aurora AV1
Visionular |
Slow (1 fps) | AV1 | Linux |
2 |
Phoenix265
Open Visual Cloud |
Slow (1 fps), Fast (30 fps) |
H.265/HEVC | v4.4, Linux |
3 |
QAV1
iQIYI Inc. |
Slow (1 fps), Fast (30 fps) |
AV1 | v2.1, Linux |
4 |
QAVS3
iQIYI Inc. |
Slow (1 fps) | AVS3 | v1.0.0, Linux |
5 |
rav1e
The rav1e contributors |
Slow (1 fps) | AV1 | 0.5.0-alpha (p20210518), Windows |
6 |
Reference x265
MulticoreWare, Inc. |
Slow (1 fps), Fast (30 fps) |
H.265/HEVC | 3.5+1-f0c1022b6, Windows |
7 |
S266
Alibaba Group |
Slow (1 fps) | H.266/VVC | v1.1, Windows |
8 |
SIF Codec
SIF Codec LLC |
Slow (1 fps) | SIF | v1.0, Windows |
9 |
SVT-HEVC
Open Visual Cloud |
Slow (1 fps), Fast (30 fps) |
H.265/HEVC | 1.5.1, Windows |
10 |
SVT-VP9
Open Visual Cloud |
Slow (1 fps), Fast (30 fps) |
VP9 | 0.3.0, Windows |
11 |
Tencent V265
Tencent |
Slow (1 fps), Fast (30 fps) |
H.265/HEVC | 1.5.0, Linux |
12 |
VVenC
Fraunhofer HHI |
Slow (1 fps) | H.266/VVC | v1.0.0, Linux |
13 |
x264
x264 project |
Slow (1 fps), Fast (30 fps) |
H.264/AVC | r3065-ae03d92, Windows |
14 |
x265
MulticoreWare, Inc. |
Slow (1 fps), Fast (30 fps) |
H.265/HEVC | 3.5+1-ce882936d, Windows |
15 |
xin26x (HEVC)
A Father (xin26x) |
Slow (1 fps), Fast (30 fps) |
H.265/HEVC | v1.0, Windows |
16 |
xin26x (VVC)
A Father (xin26x) |
Slow (1 fps), Fast (30 fps) |
VVC | v1.0, Windows |
Subjective Comparison Methodology
For subjective quality measurements we used Subjectify.us crowdsourcing platform. We involved 10,800+ participants. After deleting replies from bots we got 529,171 pairwise answers. Bradley-Terry model was used to compute global rank.
To conduct an online crowdsourced comparison, we uploaded encoded streams to Subjectify.us. For better browser compatibility we performed transcoding with x264 and CRF=16.
The platform hired study participants and showed the upload streams to them in pairs. Each pair consisted of two variants of the same test video sequence encoded by various codecs at various bitrates. Videos from each pair were presented to study participant sequentially (i.e., one after another) in full-screen mode. After viewing each pair, participants were asked to choose the video with the best visual quality. They also had the option to play the videos again or to indicate that the videos have equal visual quality. We assigned each study participant 12 pairs, including 2 hidden quality-control pairs, and each received money reward after successfully completing the task. The quality-control pairs consisted of test videos compressed by the x264 encoder at 1 Mbps and 4 Mbps. Responses from participants who failed to choose the 4 Mbps sequence for one or more quality-control questions were excluded from further consideration.
In total we collected 529,171 valid answers from 10,800+ unique participants. To convert the collected pairwise results to subjective scores, we used the Bradley-Terry model [1]. Thus, each codec run received a quality score. We then linearly interpolated these scores to get continuous rate-distortion (RD) curves, which show the relationship between the real bitrate (i.e., the actual bitrate of the encoded stream) and the quality score. Section "RD Curves" shows these curves.
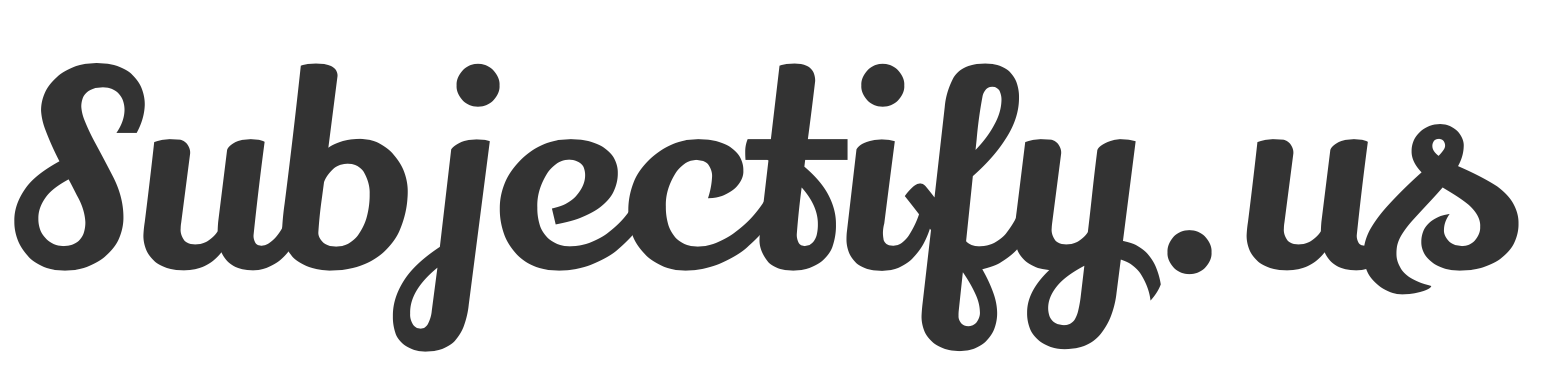
We obtained the subjective scores for this study using Subjectify.us. This platform enables researchers and developers to conduct subjective comparisons of image and video processing methods (e.g., compression, inpainting, denoising, matting, etc.) and carry out studies of human quality perception.
To conduct a study, researchers must apply the methods under comparison to a set of test videos (images), upload the results to Subjectify.us and write a task description for study participants. Subjectify.us handles all the laborious steps of a crowdsourced study: it recruits participants, presents uploaded content in a pairwise fashion, filters out responses from participants who cheat or are careless, analyzes collected results, and generates a study report with interactive plots. Thanks to the pairwise presentation, researchers need not invent a quality scale, as study participants just select the best option of the two.
The platform is optimized for comparison of large video files: it prefetches all videos assigned to a study participant and loads them into his or her device before asking the first question. Thus, even participants with a slow Internet connection won’t experience buffering events that might affect quality perception.
To try the platform in your research project, reach out to www.subjectify.us. This demo video shows an overview of the Subjectify.us workflow.
Codec Analysis and Tuning for Codec Developers and Codec Users
Computer Graphics and Multimedia Laboratory of Moscow State University:
- 17+ years working in the area of video codec analysis and tuning using objective quality metrics and subjective comparisons.
- 30+ reports of video codec comparisons and analysis (H.265, H.264, AV1, VP9, MPEG-4, MPEG-2, decoders' error recovery).
- Methods and algorithms for codec comparison and analysis development, separate codec's features and codec's options analysis.
Strong and Weak Points of Your Codec
- Deep encoder parts analysis (ME, RC on GOP, mode decision, etc).
- Weak and strong points for your encoder and complete information about encoding quality on different content types.
- Encoding Quality improvement by the pre and post filtering (including technologies licensing).
Independent Codec Estimation Comparing to Other Codecs for Different Use-cases
- Comparative analysis of your encoder and other encoders.
- We have direct contact with many codec developers.
- You will know place of your encoder between other newest well-known encoders (compare encoding quality, speed, bitrate handling, etc.).
Encoder Features Implementation Optimality Analysis
We perform encoder features effectiveness (speed/quality trade-off) analysis that could lead up to 30% increase in the speed/quality characteristics of your codec. We can help you to tune your codec and find best encoding parameters.Thanks
Special thanks to the following contributors of our previous comparisons
![]() |
![]() |
![]() |
![]() |
![]() |
![]() |
![]() |
![]() |
![]() |
![]() |
![]() |
![]() |
![]() |
![]() |
![]() |
![]() |
![]() |
![]() |
![]() |
![]() |
![]() |
![]() |
![]() |
![]() |
Contact Information
Subscribe to report updates
Other Materials
Video resources:
Server size: 8069 files, 1215Mb (Server statistics)
Project updated by
Server Team and
MSU Video Group
Project sponsored by YUVsoft Corp.
Project supported by MSU Graphics & Media Lab